Summary โ
Together with our global community of contributors, GreptimeDB continues to evolve and flourish as a growing open-source project. We are grateful to each and every one of you.
Below are the highlights among recent commits:
Introduced Flow Engine to provide continuous aggregation capabilities.
Expressions can be used in Range Query, such as
RANGE (INTERVAL '2' day - INTERVAL '1' day)
.Support for using
EXPLAIN ANALYZE
in distributed mode to analyze query execution costs.
Contributors โ
For the past two weeks, our community has been super active with a total of 86 PRs merged. 12 PRs from 8 individual contributors merged successfully, and lots are pending to be merged.
Congrats on becoming our most active contributors in the past 2 weeks:
๐ Welcome contributor @groobyming @ltratt @tizee join to the community as the new individual contributor, and congratulations on successfully merging their first PR, more PRs are waiting to be merged.
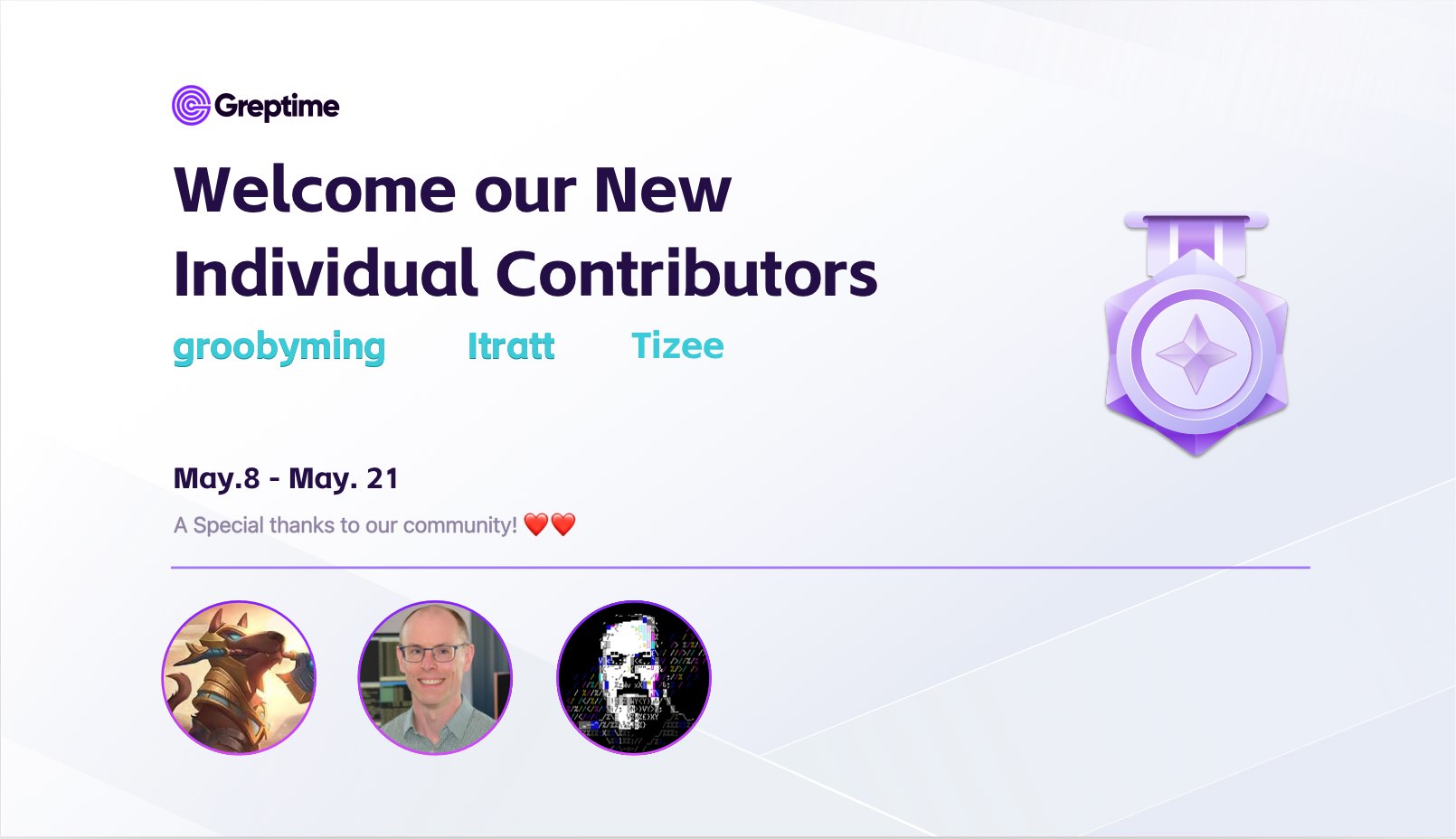
A big THANK YOU to all our members and contributors! It is people like you who are making GreptimeDB a great product. Let's build an even greater community together.
Highlights of Recent PRs โ
db#3823 Using expressions in Range Query โ
Now, the Range Query has new support for the following features:
Supporting Interval type calculations in the Range and Align parameters. For example, you can use
(INTERVAL '2' day - INTERVAL '1' day)
to compute time intervals.Specifying the time origin for alignment using expressions. For instance,
(now() - INTERVAL '1' hour)
can be used to align the query to one hour before the current time.
SELECT
ts,
min(val) RANGE (INTERVAL '2' day - INTERVAL '1' day)
FROM
host
ALIGN (INTERVAL '2' day - INTERVAL '1' day)
TO (now() - INTERVAL '1' hour)
ORDER BY ts;
These enhancements allow users to define time ranges and alignment points more flexibly, enabling more precise time-series data analysis.
Range Query is an extension syntax of GreptimeDB. For more information: https://docs.greptime.com/reference/sql/range
db#3908 Supporting EXPLAIN ANALYZE in a distributed mode โ
In distributed mode, GreptimeDB now supports EXPLAIN ANALYZE
, which executes the corresponding SQL statement. By running EXPLAIN ANALYZE
, users can gain detailed insights into the performance of distributed queries, including execution time and resource utilization metrics. This feature is crucial for optimizing and troubleshooting complex queries in distributed environments.
explain analyze SELECT count(*) FROM system_metrics;
+-------+------+---------------------------------------------------------------------------------------------------------------------------------------------------------------------------------+
| stage | node | plan |
+-------+------+---------------------------------------------------------------------------------------------------------------------------------------------------------------------------------+
| 0 | 0 | MergeScanExec: peers=[4402341478400(1025, 0), ] metrics=[output_rows: 1, greptime_exec_read_cost: 0, ready_time: 6352264, finish_time: 7509279, first_consume_time: 7165836, ] |
| | | |
| 1 | 0 | AggregateExec: mode=Final, gby=[], aggr=[COUNT(greptime.public.system_metrics.ts)] metrics=[output_rows: 1, elapsed_compute: 108029, ] |
| | | CoalescePartitionsExec metrics=[output_rows: 32, elapsed_compute: 83055, ] |
| | | AggregateExec: mode=Partial, gby=[], aggr=[COUNT(greptime.public.system_metrics.ts)] metrics=[output_rows: 32, elapsed_compute: 334913, ] |
| | | RepartitionExec: partitioning=RoundRobinBatch(32), input_partitions=1 metrics=[repart_time: 1, fetch_time: 441565, send_time: 30325, ] |
| | | StreamScanAdapter { stream: "<SendableRecordBatchStream>" } metrics=[output_rows: 3, mem_used: 24, ] |
| | | |
| | | Total rows: 1 |
+-------+------+---------------------------------------------------------------------------------------------------------------------------------------------------------------------------------+
db#3923 Migrated the dependency from orc-rs to datafusion-orc โ
Earlier, we donated orc-rs to the datafusion-contrib community and maintained it together with the community. Recently, the main repository's dependency on orc-rs has been migrated to datafusion-orc.
db#3932 Fuzz testing now includes validation of inserted data โ
In previous work, we added fuzz testing for data writes. In this PR, we further enhanced it by adding validation for the data that has been written to ensure it meets the expected criteria.
Good First Issue โ
db#3997 Conduct fuzz testing for GreptimeDB clusters using shared storage โ
In db#3967, we ran GreptimeDB clusters and fuzz tests in CI. To better simulate real-world usage, we want to run fuzz testing for GreptimeDB clusters using shared storage in CI.
Keywords: CI
Difficulty: Medium
db#3973 Add fuzz testing for the column type change feature โ
In db#3517, we supported altering the column data type. We need to add corresponding fuzz tests for this feature.
Keywords: Fuzz testing
Difficulty: Medium
db#3884 Remove unnecessary traits and wrapper types from the query crate โ
The manifest doesn't have any checksum for data validation. We need a way to do the checksum validation for region manifests. A possible way is to save the checksum as the part of manifest file name, for example, 000000000001-{checksum}.json
.
Most implementations simply forward requests to Datafusion. Since we are highly coupled with Datafusion and have no plans to support another query engine, we can remove these types.
Keywords: Refactoring
Difficulty: Simple
About Greptime โ
Greptime offers industry-leading time series database products and solutions to empower IoT and Observability scenarios, enabling enterprises to uncover valuable insights from their data with less time, complexity, and cost.
GreptimeDB is an open-source, high-performance time-series database offering unified storage and analysis for metrics, logs, and events. Try it out instantly with GreptimeCloud, a fully-managed DBaaS solutionโno deployment needed!
The Edge-Cloud Integrated Solution combines multimodal edge databases with cloud-based GreptimeDB to optimize IoT edge scenarios, cutting costs while boosting data performance.
Star us on GitHub or join GreptimeDB Community on Slack to get connected.